Mistakes businesses makes deploying AI enterprise apps
The Fast Company Impact Council is an invitation-only membership community of leaders, experts, executives, and entrepreneurs who share their insights with our audience. Members pay annual dues for access to peer learning, thought leadership opportunities, events and more. Many companies forget AI-powered enterprise applications are just business apps at the end of the day. The reality is, AI is simply another arrow in our quiver, albeit incredibly more powerful. But what IT has done since generative AI exploded on the scene is frantically rush to deploy any and all possible applications, causing massive confusion and huge resource wastes, without delivering much business value. The process of firing arrows at the target (increased business value) has stayed the same, just like the goal of hitting a bullseye. But many businesses miss the mark, trying to create significant and oftentimes unrealistic returns. How to generate more value with AI To be fair, the urgency is real, particularly as the next big target arises. Gartner predicts, “By 2028, 33% of enterprise software application will include agentic AI, up from less than 1% in 2024, enabling 15% of day-to-day work decisions to be made autonomously.” Everyone wants to fire with precision. They’re just unsure where to aim. At our recent Insight Amplify technical conference, I sat down with a handful of fellow tech executives. We discussed our experiences rolling out AI apps, and we agreed that most successful use cases so far have been internal-facing as a proving ground to master the technology. Slowly but surely, the hope is it shows its mettle in customer-facing applications. Everyone agrees this is uncharted territory. I not-so-innocently asked a few follow-up questions, such as how they went about finding workloads. They each described the broad strokes, and as I suspected, their processes were remarkably similar. There’s much more nuance to it, but generally they followed these steps: Identify the business problem, ideally in partnership between the business line and IT team. Build a minimum viable product and, with a subset of capabilities, deploy the app to see if it is appropriate from functional and practical standpoints. Evaluate the return on investment to ensure it makes sense financially. Expand it for widespread use with the necessary cost and security controls and backlogged features. These are the same proven steps to build a “traditional” app—yet all too often, adding the shiny AI component blinds us from the fundamentals. When I pointed out the similarities, it was like a light bulb flipped on. They hadn’t thought about it like that. Unfortunately, many companies struggle to reach that “Eureka” moment in app development and make a few common missteps along the way. For example: Misstep #1: Developing AI apps yourself The first step is critical. Without a clear business problem, is there a point to pouring resources into an app? Even if a company has a solution, they need to step back and determine if they can deliver it. I often see clients trying to reinvent the wheel when they’re unequipped to do so. If a company’s business isn’t writing software, they should focus on their core business instead. There’s a reason why auto manufacturers don’t make the lights that go into cars. They simply buy and put them into what they build. That’s the mentality businesses should have with AI—relying on partners with the specialized knowledge to guide them through this evolving landscape. This isn’t to say buying off the shelf is always the answer. Sometimes, the need is unique enough or rooted in specific business processes where developing custom AI-powered solutions makes sense. But first look for someone doing that as their business. If you can’t find them, then—and only then—create your own. Misstep #2: Improperly preparing your data I sometimes joke that clients have data swamps, not data lakes. Poor data quality is a significant gap in many organizations. It can be terribly organized and inadequately secured across different sources, costing companies 15-25% of their revenue, according to MIT Sloan Management Review. The key to unlocking data’s immense value lies in organizing and normalizing it in one place, but most data is siloed across various locations based on its functions. While a small subset may seem manageable, this can mask underlying issues that arise once you deploy an app to a larger end-user community if your data isn’t properly cleaned. This will be problematic whether you buy off the shelf or develop yourself. Data mastery is fundamental to driving any outcome. Misstep #3: Locking yourself in My colleagues are right: This is new territory. While basic app development steps remain the same, a rapidly evolving sector introduces countless variables to consider. Even without GenAI, changes would still occur at breakneck
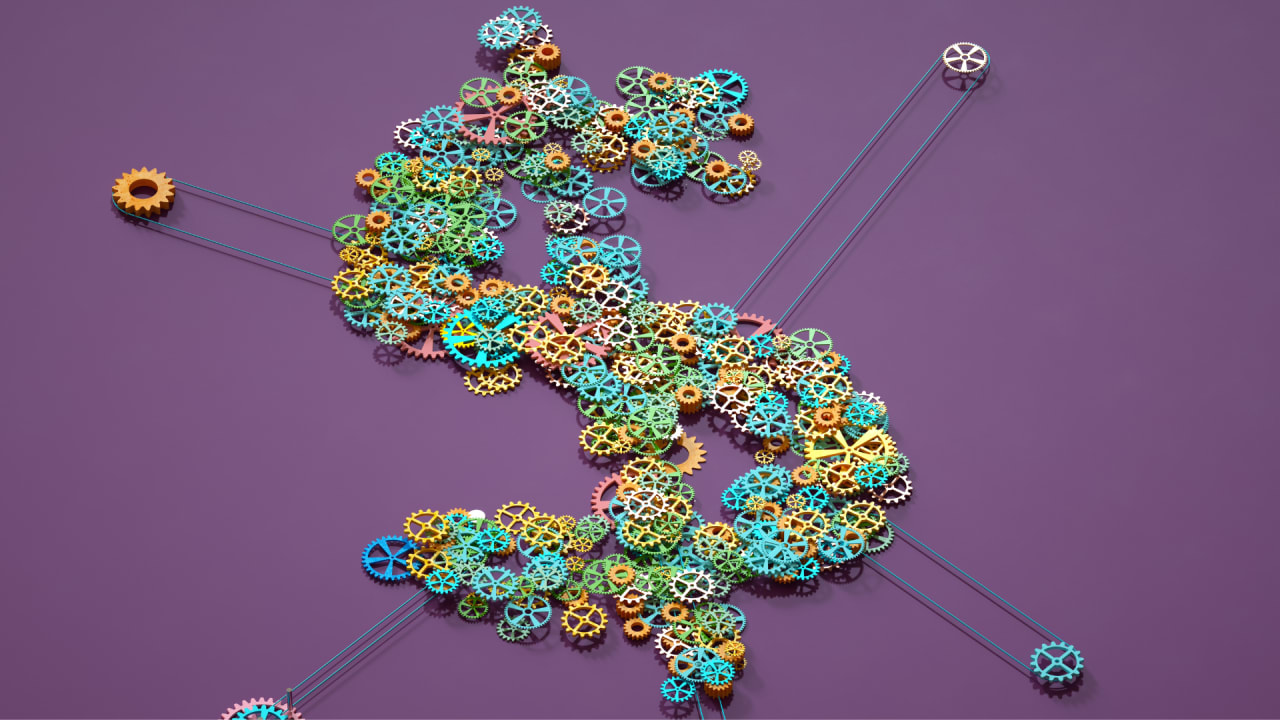
The Fast Company Impact Council is an invitation-only membership community of leaders, experts, executives, and entrepreneurs who share their insights with our audience. Members pay annual dues for access to peer learning, thought leadership opportunities, events and more.
Many companies forget AI-powered enterprise applications are just business apps at the end of the day. The reality is, AI is simply another arrow in our quiver, albeit incredibly more powerful. But what IT has done since generative AI exploded on the scene is frantically rush to deploy any and all possible applications, causing massive confusion and huge resource wastes, without delivering much business value.
The process of firing arrows at the target (increased business value) has stayed the same, just like the goal of hitting a bullseye. But many businesses miss the mark, trying to create significant and oftentimes unrealistic returns.
How to generate more value with AI
To be fair, the urgency is real, particularly as the next big target arises. Gartner predicts, “By 2028, 33% of enterprise software application will include agentic AI, up from less than 1% in 2024, enabling 15% of day-to-day work decisions to be made autonomously.”
Everyone wants to fire with precision. They’re just unsure where to aim.
At our recent Insight Amplify technical conference, I sat down with a handful of fellow tech executives. We discussed our experiences rolling out AI apps, and we agreed that most successful use cases so far have been internal-facing as a proving ground to master the technology. Slowly but surely, the hope is it shows its mettle in customer-facing applications.
Everyone agrees this is uncharted territory.
I not-so-innocently asked a few follow-up questions, such as how they went about finding workloads. They each described the broad strokes, and as I suspected, their processes were remarkably similar.
There’s much more nuance to it, but generally they followed these steps:
- Identify the business problem, ideally in partnership between the business line and IT team.
- Build a minimum viable product and, with a subset of capabilities, deploy the app to see if it is appropriate from functional and practical standpoints.
- Evaluate the return on investment to ensure it makes sense financially.
- Expand it for widespread use with the necessary cost and security controls and backlogged features.
These are the same proven steps to build a “traditional” app—yet all too often, adding the shiny AI component blinds us from the fundamentals. When I pointed out the similarities, it was like a light bulb flipped on. They hadn’t thought about it like that. Unfortunately, many companies struggle to reach that “Eureka” moment in app development and make a few common missteps along the way. For example:
Misstep #1: Developing AI apps yourself
The first step is critical. Without a clear business problem, is there a point to pouring resources into an app? Even if a company has a solution, they need to step back and determine if they can deliver it. I often see clients trying to reinvent the wheel when they’re unequipped to do so.
If a company’s business isn’t writing software, they should focus on their core business instead. There’s a reason why auto manufacturers don’t make the lights that go into cars. They simply buy and put them into what they build. That’s the mentality businesses should have with AI—relying on partners with the specialized knowledge to guide them through this evolving landscape.
This isn’t to say buying off the shelf is always the answer. Sometimes, the need is unique enough or rooted in specific business processes where developing custom AI-powered solutions makes sense. But first look for someone doing that as their business. If you can’t find them, then—and only then—create your own.
Misstep #2: Improperly preparing your data
I sometimes joke that clients have data swamps, not data lakes. Poor data quality is a significant gap in many organizations. It can be terribly organized and inadequately secured across different sources, costing companies 15-25% of their revenue, according to MIT Sloan Management Review.
The key to unlocking data’s immense value lies in organizing and normalizing it in one place, but most data is siloed across various locations based on its functions. While a small subset may seem manageable, this can mask underlying issues that arise once you deploy an app to a larger end-user community if your data isn’t properly cleaned. This will be problematic whether you buy off the shelf or develop yourself. Data mastery is fundamental to driving any outcome.
Misstep #3: Locking yourself in
My colleagues are right: This is new territory. While basic app development steps remain the same, a rapidly evolving sector introduces countless variables to consider. Even without GenAI, changes would still occur at breakneck speeds. Welcome to IT.
Among all the AI hype, what is just noise you can ignore? What are legitimate signs of the frenetic activity around us?
I guide clients through these types of questions all the time. Developing AI, they might invest too much capital in on-premises solutions, lock themselves into a specific cloud provider, or partner with an independent software vendor that’s a darling today but dead in six months.
Given technology’s rapid pace, it’s crucial to stay flexible. You’ll need to pivot eventually. Locking yourself into a category, location, vendor, or similar commitment is extremely risky.
The stumbling block that so many struggle with is they don’t yet have enough muscle memory working with AI to unlock its full potential. In the absence of certainty, what should be logical is to do what’s familiar—what’s worked before:
- Stick to your strengths as a business.
- Stick to proven app-development processes if that is, in fact, your business.
- If not: Stay the course with trusted partners who have that expertise.
In other words, don’t overthink things. It’s AI—not rocket science, unless that’s the app you need.
If you’re unsure where to begin, work with a solutions provider with proven success delivering agentic, generative, and traditional AI applications. With a few reps under your belt, you’ll be locked in to hit future targets.
Juan Orlandini is CTO, North America of Insight Enterprises.