Why AI needs zkML: the missing puzzle piece to AI accountability
A new approach to contemporary AI accountability challenges.
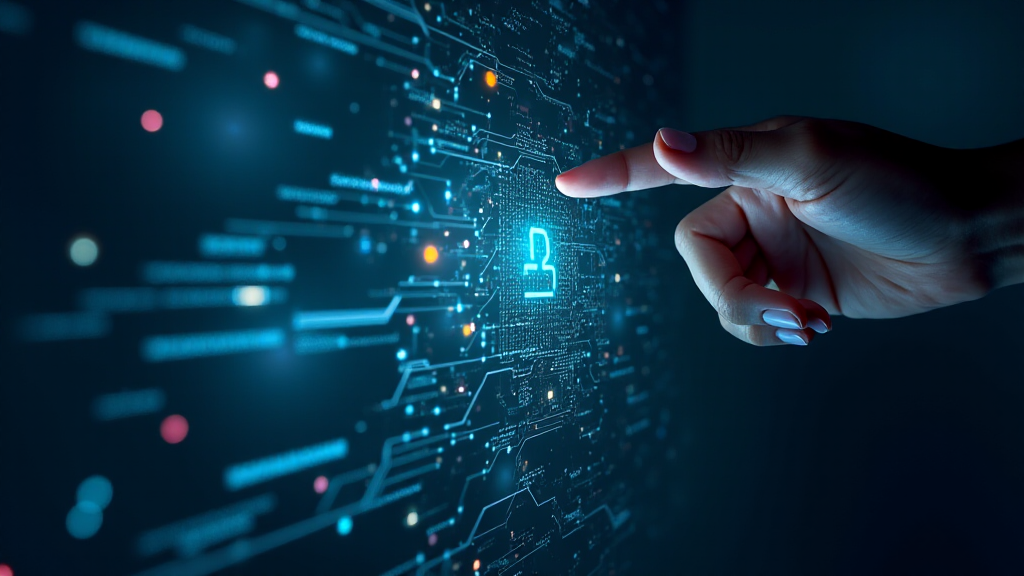
From DeepSeek to Anthropic’s Computer Use and ChatGPT’s ‘Operator,’ AI tools have taken the world by storm, and this may be just the beginning. Yet, as AI agents debut with remarkable capabilities, a fundamental question remains: how do we verify their outputs?
The AI race has unlocked groundbreaking innovations, but as development surges ahead, key questions around verifiability remain unresolved. Without built-in trust mechanisms, AI’s long-term scalability — and the investments fueling it — face growing risks.
The Asymmetry of AI Development vs. AI Accountability
Today, AI development is incentivized for speed and capability, while accountability mechanisms lag behind. This dynamic creates a fundamental imbalance: verifiability lacks the attention, funding and resources needed to keep pace with AI progress, leaving outputs unproven and susceptible to manipulation. The result is a flood of AI solutions deployed at scale, often without the safety controls needed to mitigate risks like misinformation, privacy breaches and cybersecurity vulnerabilities.
This gap will become more evident as AI continues to integrate into critical industries. Companies developing AI models are making remarkable strides — but without parallel advancements in verification, trust in AI risks being eroded. Organizations that embed accountability from the outset won’t just mitigate future risks; they’ll gain a competitive advantage in a landscape where trust will define long-term adoption.
AI’s rapid adoption is an incredible force for innovation, but with that momentum comes the challenge of ensuring robust verification without slowing progress. Rather than leaving critical concerns for later, we provide a seamless path to integrate verifiability from the start — so developers and industry leaders can move full speed ahead with confidence. The current AI gold rush has unlocked massive opportunities, and by closing the gap between capability and accountability, we ensure that this momentum not only continues but strengthens for the long term.
Verifiability as a Catalyst for AI’s Future
Recently, many were surprised when one of the largest tech companies in the world pulled the plug on its AI features. But as AI capabilities expand, should we really be caught off guard when verification challenges surface? As AI continues to scale, the ability to prove its trustworthiness will determine whether public confidence grows or diminishes.
Recent surveys indicate that skepticism is rising, with a significant portion of users expressing concern over AI’s reliability. The next evolution of AI requires accountability to grow in tandem with development, ensuring trust scales with innovation.
The future of AI needs to be reframed: The question is no longer just ‘Can AI do this or that?’ but rather ‘Can we trust AI’s outputs?’ By embedding trust and verification into AI’s foundations, the industry can ensure AI adoption continues to expand with confidence.
But to return to the fundamental question at hand: how? More precisely, how do you know if the information generated from AI is accurate? How can the privacy and confidentiality of that information be verified? Anyone using ChatGPT, Copilot, Perplexity or Claude, among countless others, has faced these questions. Addressing them requires leveraging the latest advancements in cryptographic verification.
Enter zkML: A Framework for AI Trust
AI’s ability to generate complex outputs is growing exponentially, but verifying the accuracy, security and trustworthiness of these outputs remains an open challenge. This is where zero-knowledge machine learning (zkML) presents a breakthrough solution.
Zero-knowledge proofs (ZKPs), originally developed for cryptographic security, provide a way to prove the validity of an AI-generated output without revealing the underlying data or model details. By applying these techniques to machine learning, zkML ensures that AI-generated outputs are produced as expected while preserving privacy and integrity.
Inference generated using zkML confirms that AI models operate as intended, while verifiable AI training ensures that the training data remains untampered. Additionally, private input protection allows AI to be leveraged securely without exposing sensitive information, and compliant, confidential AI helps meet regulatory requirements while preserving data confidentiality. This means AI systems can prove their outputs — without disclosing the full details of their processes, including model weights.
Unlike traditional verification methods that rely on centralized oversight or controlled environments, zkML enables decentralized, trustless verification. This allows AI developers to demonstrate the authenticity of their models without requiring external trust assumptions, paving the way for scalable and transparent AI verification.
The Future of AI Trust Hinges on Verifiability
AI’s credibility hinges on its ability to prove its outputs are trustworthy. The industry has an opportunity to integrate verifiability now — before trust erodes.
A future where AI operates without trust mechanisms will struggle to scale sustainably. By integrating cryptographic verification techniques like ZKPs, we can create an AI ecosystem where transparency and accountability are built in, not an afterthought.
Verifiable AI is more than a theoretical solution; it’s the next frontier of AI innovation. The shift toward verifiable AI is not only necessary — it’s the next step in ensuring AI’s long-term success. The time to act is now.
We've listed the best encryption software.
This article was produced as part of TechRadarPro's Expert Insights channel where we feature the best and brightest minds in the technology industry today. The views expressed here are those of the author and are not necessarily those of TechRadarPro or Future plc. If you are interested in contributing find out more here: https://www.techradar.com/news/submit-your-story-to-techradar-pro